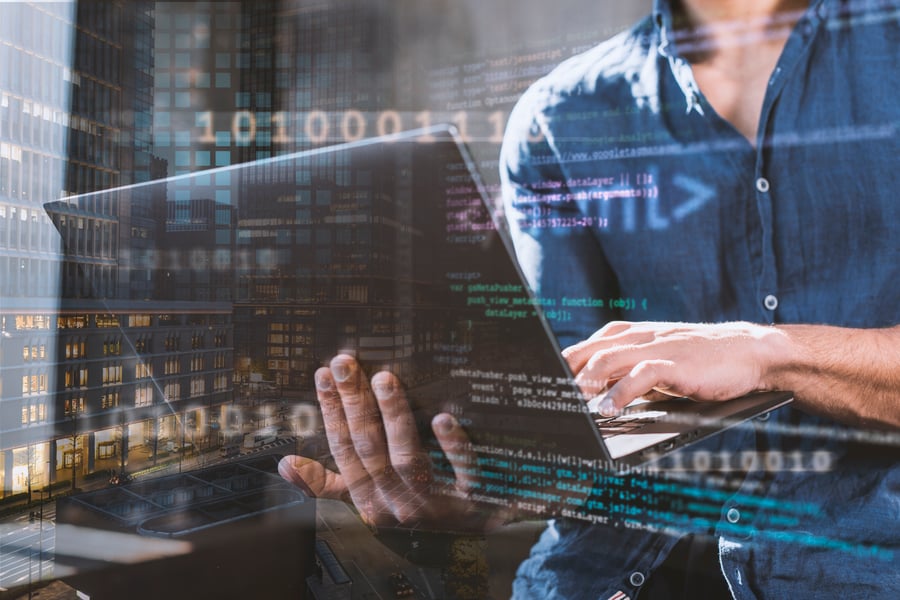
The Growing Challenge of Big Data
The Necessity of Automated DataOps
Automated DataOps offers a scalable, efficient solution to these challenges. By automating data pipeline operations, businesses can address several critical issues:
- Data Integration and Quality: Automated DataOps simplifies integrating data from multiple sources and maintaining data quality at scale, ensuring accurate analysis and decision-making.
- Scalability: As data volume and complexity grow, automated DataOps facilitates the scaling of data pipelines, enabling businesses to manage their data more effectively without compromising performance.
- Security and Privacy: Automation helps enforce data security and privacy measures, streamlining access controls and regulatory compliance.
- Monitoring, Maintenance, and Governance: Automated processes reduce the resource requirements for continuous monitoring, maintenance, and governance, ensuring data is managed appropriately throughout its lifecycle
Overcoming Inefficiencies with Automation
The manual management of data ingestion, transformation, and version control is fraught with inefficiencies. Automated DataOps directly addresses these challenges by streamlining operations and reducing potential errors. This not only enhances performance but also significantly reduces operational costs.
Leveraging AWS Tools for Automated DataOps
Amazon Web Services (AWS) offers a robust suite of tools designed to facilitate the implementation of automated DataOps, enabling businesses to manage high-volume data efficiently. By leveraging these tools, organizations can significantly improve their data management processes, from ingestion to analytics.
- Amazon S3 & AWS Glue: Amazon Simple Storage Service (S3) provides scalable object storage, ideal for storing and retrieving data. When combined with AWS Glue, a serverless data integration service, businesses can quickly discover, prepare, and combine data for analytics, machine learning, and application development.
- Amazon Redshift: For data warehousing, Amazon Redshift offers a fast, scalable platform that simplifies data analysis across vast datasets using standard SQL. Its seamless integration with data lakes and operational databases enables comprehensive analytics solutions.
- AWS Data Pipeline: AWS Data Pipeline automates the movement and transformation of data. With its highly reliable and scalable architecture, businesses can quickly process and move data between different AWS compute and storage services and on-premises data sources.
- Amazon Athena: Amazon Athena allows users to directly query data in Amazon S3 using standard SQL, making it easier to analyze unstructured data without moving it into a separate analytics database. This service supports a serverless architecture, enabling scaling without infrastructure management.
- AWS Lambda: For operationalizing automated DataOps workflows, AWS Lambda supports running code in response to triggers such as changes in data, system state, or user actions without provisioning or managing servers. This facilitates real-time data processing and integration tasks.
Integrating AWS Tools into Existing Workflows
Integrating AWS tools into existing data management workflows can significantly enhance scalability and minimize errors. Starting with a pilot project, such as automating data ingestion into Amazon S3 or setting up a data processing workflow with AWS Lambda, allows businesses to adopt automated DataOps practices gradually. Key to success is ensuring that the selected AWS tools align with the organization's specific data management needs and that staff are trained to leverage these tools effectively.
Real-World Success Stories
Integrating AWS tools for automated DataOps has proven transformative for industries grappling with high-volume data management. A notable example is Adani Power, which significantly enhanced operational efficiency in collaboration with Mactores and AWS by migrating to Amazon Timestream for real-time data analysis, employing Amazon SageMaker for machine learning models, and utilizing Amazon Forecast for accurate load forecasting. Automation of data processing and analysis, resulting in increased efficiency, reduced manual effort, and improved accuracy of data analysis. This strategic adoption streamlined data management across vast sensor networks and equipment and facilitated real-time monitoring and decision-making, reducing operational costs, improving system reliability, and increasing revenue. This case study underscores the potential of automated DataOps, powered by AWS technologies, to drive substantial improvements in business operations and outcomes. Read more about the case study here.
Looking Ahead: The Future of Data Management
The future of high-volume data management is inherently tied to the advancements in automated DataOps. Emerging technologies, such as artificial intelligence (AI) and machine learning (ML), are set to automate data operations further, making achieving even greater efficiencies and insights possible. As data privacy and governance continue to gain importance, automated DataOps will play a crucial role in ensuring compliance while enabling businesses to leverage their data assets fully.
Conclusion
Automated DataOps represents a significant shift in the landscape of data management. By embracing this technology, businesses can overcome the challenges of high-volume data management, achieve scalability, improve performance, and reduce errors. As we look to the future, the continued evolution of automated DataOps promises to offer even more opportunities for businesses to harness the power of their data for sustainable success. The time to adopt automated DataOps is now, and businesses that take the lead in this transition will be well-positioned to thrive in the data-driven world.
If you want to leverage automated DataOps in scaling your business, then let's have a chat.