Automated MLOps
Improve operational efficiencies, automate ML workflows and accelerate prototyping.
When to use
Automated MLOps solutions?
If you are looking to utilize AI/ML to revolutionize your business at scale, then you need to implement a MLOps solution that not only gives your organization a one-stop solution for your machine learning engineers, data scientists, and data engineering teams.
With the right automated MLOps pipelines, you can establish a data architecture that facilitates data analysis, enables rapid prototyping, productionizes the NL pipeline and deploys the ML models.
If you are looking to leverage your business’ various data sources and uncover new business value and growth, machine learning automation can help create a robust pipeline between disparate data sources and accessing the insights locked within that data.
The Mactores MLOps solution caters to customers with simple to complex AI/ML use cases by adopting cutting edge CI/CD techniques to help data scientists achieve frictionless automation for their experiments and production deployment of the models.
Streamline the challenges in operationalizing ML models by creating an automated medium for data scientists, software engineering, operations, and business to create, deploy and operate ML models.
Create structure and transparency in the end-to-end ML pipeline and align your ML models with your business’ needs and regulatory requirements with an automated MLOps pipeline.
99%
reduction of the time required to productionalize the model
60%
additional efficiency to measure model quality monitoring
What does
Mactores Automated MLOps deliver:
The Mactores Automated MLOps solution creates a robust pipeline between the end users and those tasked with managing the data.
This pipeline is the conduit between piles of data and access to the insights it holds for those in charge of the various business units at an organization.
With over 10 years of experience, we can help establish machine learning automation for your business, which will result in:
-
Automation of data validation, data preparation, training ML models, model evaluation, and model validation.
-
Ensuring appropriate metadata management to help with data and artifacts lineage, reproducibility, and comparison.
-
Streamlining the challenges in operationalizing ML models.
Our MLOps process is focused on outcomes and not just output. Our well-defined process of deliberation and envisioning sessions help customers focus on business objectives.
By working with Mactores, you can achieve:
-
Substantially improved business value
-
Faster time to market
-
A competitive advantage
-
A comprehensive managed solution
The Mactores
Process
Mactores utilizes a wide range of valuable tools to explore possible automation solutions and business outcomes. Through exploration and validation of existing data, we can help prevent your AI/ML project from failing.
Our comprehensive MLOps process consists of eight outcome-oriented steps:
1- Envisioning Session:
Our AI experts and your team explore possible automation solutions and business outcomes using AI/ML advancements and provide sufficient and necessary inputs to proceed to the next stage.
2- Vision Document:
We conduct a hyper-focused session on the outcomes of your ML Solution and build a vision document covering every aspect of the final solution which needs to be addressed in the due process.
3- Data Exploration and Validation:
Our team starts building cohorts of the data available and provides validation to proceed with further steps. Most AI/ML initiatives fail due to insufficient data or improper identification of business problems based on the data. We act as a gatekeeper to ensure your AI/ML project does not fail.
4- Data Preparation:
Our AI/Ml experts start cleaning the data, normalize the features, and perform tasks related to encoding features in your data to start training initial sample models.
5- Model Selection:
Our data scientists will evaluate and select the best performing models to solve the problem.
6- Training and Validation:
Once at least three models are selected, our team will train and run validations on all the options available before proceeding to the final model selection. This is an iterative process and sometimes takes multiple weeks to reach the business objective defined in the Vision Document.
7- Production Deployment:
After the final model is selected, our Data Operation Team will finalize the production deployment of the model for production use and integrate with business solutions.
8- Post-Production Training:
We will set up your ML pipeline to continuously evolve and improve the results of the solution we have built. We will identify the periodicity of the training required by your business problem and data available. We will also set up the pipeline to automate the training, validation, and production deployment of improved models.
Key Customer Pain Points
Our goal is to help your business improve operational efficiency, create models in production faster, and accelerate experimentation speed.
We can help you avoid the most common issues:
- Paying for expensive license renewals every year.
- Unable to scale your databases, creating the challenge of managing data at scale, originating from multiple databases and then processing all the data with the flexibility to process extreme usage demands over various peaks and valleys of the business cycle.
- Unable to make your database globally available.
- Do not have a high availability of your database.
- You’ve chosen the wrong type of database for the right use case.
- You are trying to reduce your infrastructure costs.
- You are trying to solve your maintenance challenges.
- You want to minimize the workload on lean and overworked teams.
You can create scalable infrastructure, identify key data science processes and identify the multiple roles and responsibilities required to develop a holistic approach that addresses your access to transformational data insights.
Ideal uses for Automated MLOps
Unify the release cycle for machine learning and software application release
Implementing Model Versioning, A/B Tesing and Model monitoring
Building Ethical AI Framework
Implement CRISP Machine learning model management framework
Model Governance
Data Analysis and Environment Management
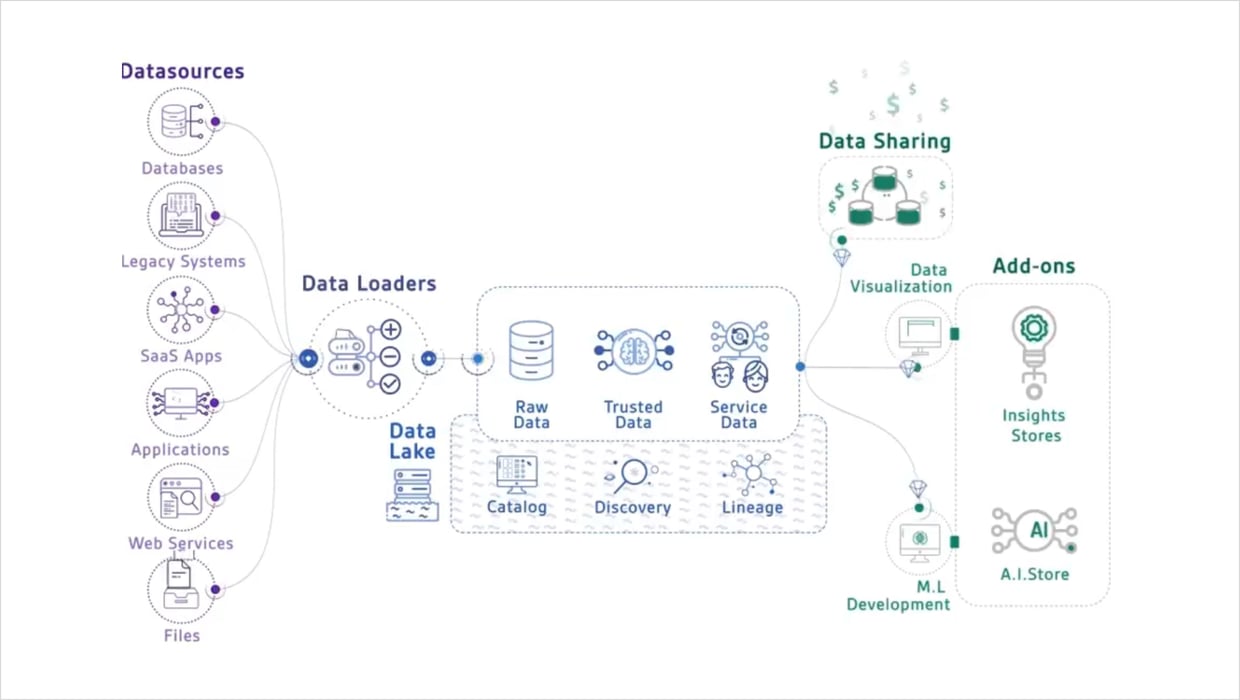
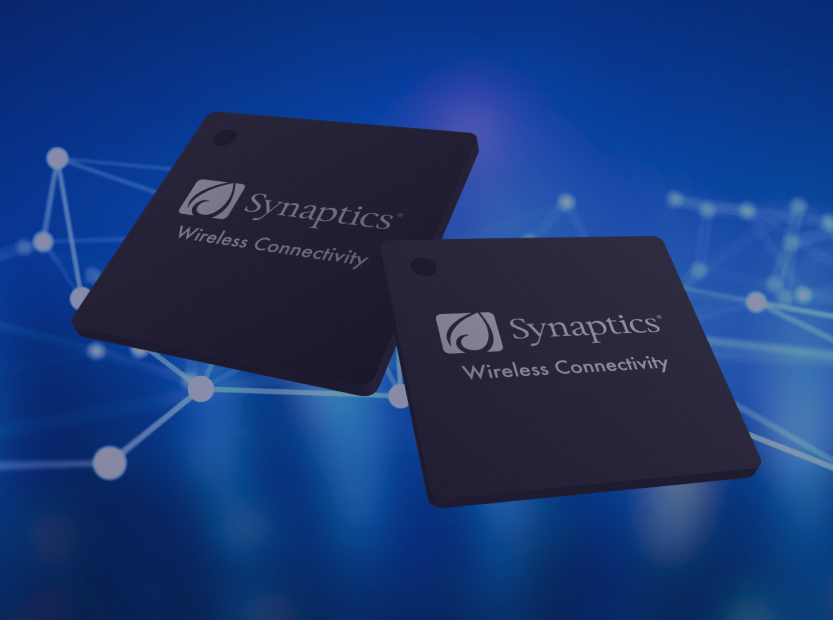
Case Study
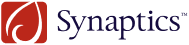
AI-backed operational data lake for Synaptics
Synaptics was challenged to operate and manage an EDA workload for multiple designs and testing teams using an HPC solution. Mactores Data Lake and AI solutions improved their design jobs' efficiency by 75% and throughput of the HPC solution by 40%.
AWS Validated
Competencies & Service Deliveries
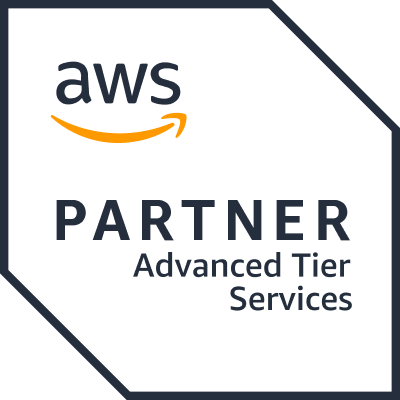
Deep technical expertise with multiple partner program validations and demonstrated success working with a large number of customers at scale.
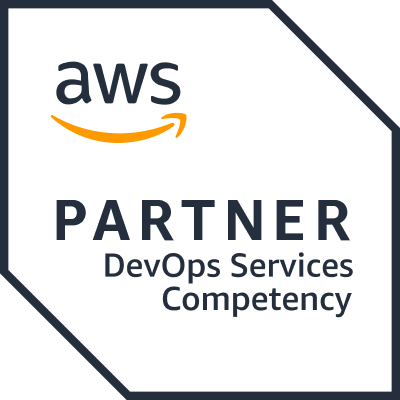
Mactores demonstrated expertise in delivering an agile culture and processes by adopting best practices for infrastructure such as code, CI/CD, security, monitoring, and logging.
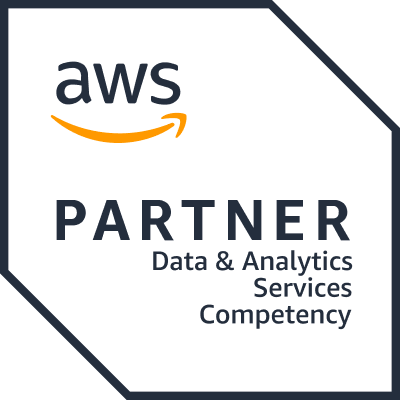
Mactores demonstrated success in helping 100+ customers transform data into value by evaluating and using the tools and best practices for collecting, storing, governing, and analyzing data at any scale.
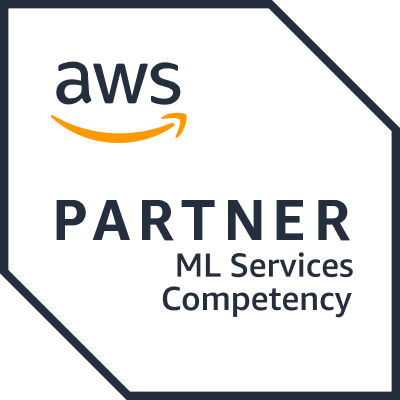
Mactores demonstrated expertise in delivering applications and analytics workloads transformation while moving to AWS to reduce cost, increase agility, and improve security.
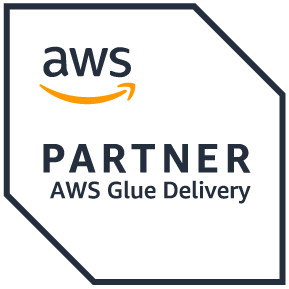
Achieving the AWS Glue Service Delivery badge highlights Mactores’ expertise in delivering seamless, scalable data integration and ETL solutions, empowering businesses to unlock insights and drive innovation with optimized data pipelines.
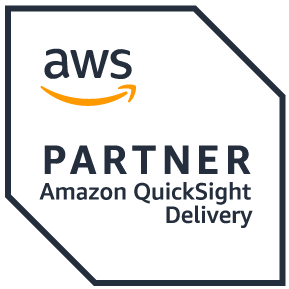
As an AWS QuickSight Delivery Partner, Mactores empowers businesses with scalable, AI-driven BI solutions. These solutions enable real-time insights, enhanced decision-making, and seamless analytics integration for faster, data-driven outcomes.
From Our Experts
Discover advice, support, tips, and a no-nonsense approach to problem solving.