The data-driven enterprise of the future embeds analytics insights into every decision, interaction, and business operation. From prescriptive and predictive analytics to
advanced machine learning models that process large volumes of real-time data streams at scale, organizations of all sizes and industry verticals rely on a highly-available, secure, and flexible data lake architectural framework for their cloud-based analytics and data platform.
Are you up to date on the latest data analytics trends? Let’s look at a few trends in
data analytics that cross through multiple industries and see how modernizing your data can help your business compete.
Growing Use Cases for Data Management and Analytics
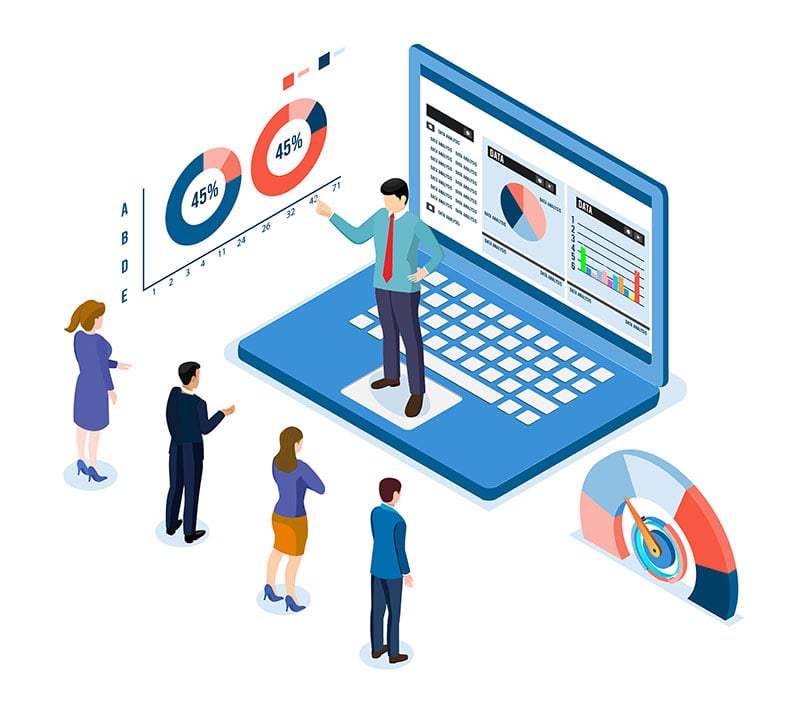
- Descriptive Analytics: Answering basic questions about historical data: what it is, when and where it happened, and in what quantity.
- Diagnostic Analytics: Understanding why this happened – gaining knowledge about the underlying characteristics.
- Predictive Analytics: What might happen in the future? Using historical evidence to predict the future.
- Prescriptive Analytics: Finding the best action plan based on contextual and recorded knowledge.
All types of data analytics help organizations of all industry verticals make better decisions faster and establish a safe and secure operating environment in the digital ecosystem. Also, find unprecedented new revenue opportunities and business models and capitalize on untapped intelligence from the vast pool of data assets already available to these organizations. With the right data governance strategy enabled by the data lake architecture and flexible IAM controls aligned with the organizational policy, data assets are available for all data consumers and business functions within a secure and fully compliant environment. Users do not spend unnecessary resources processing and maintaining multiple versions of data assets to meet their analytics requirements. The cost of running large-scale analytics is also reduced on the modern data platform as the data orchestration process is streamlined and flexible to meet changing business needs. Everyday use cases include
- Process Optimization: Identifying performance bottlenecks and optimizing business processes for maximum resource utilization.
- Business Unit Analytics: Every business unit ranging from finance and operations to marketing, sales, and customer support, can leverage advanced analytics capabilities to improve decision-making, identify performance bottlenecks and optimize business processes.
- Network Performance: Analyzing large volumes of real-time network traffic streams and log data to proactively evaluate network performance and automating control actions to maintain SLA metrics performance.
- Cybersecurity: Analyzing the performance of the IT network, apps, and systems, data transfers, and access trends to discover abnormal behavior such as network infringement and data breach incidents.
- Predictive Support and Maintenance: Analyzing the performance of systems and machines to proactively schedule maintenance activities and upgrades that improve service dependability.
To achieve these goals, the future of data analytics will inherently depend on the ability to process and deliver data analytics in real-time, embedding data into every step of your decision-control processes and developing a modern platform that streamlines the end-to-end analytics process – from data collection, acquisition, and integration to data transformation, movement, and processing. Flexible purpose-built data stores that integrate multiple data consumers and data producers while maintaining stringent data governance, security, and quality controls will be essential, as distributed business functions rely on numerous real-time data streams and third-party SaaS analytics services for a variety of analytics use cases. How can an organization scale its data platform to achieve these goals without facing security, compliance, or technology performance issues?
Hybrid Cloud Solutions and Cloud Computing
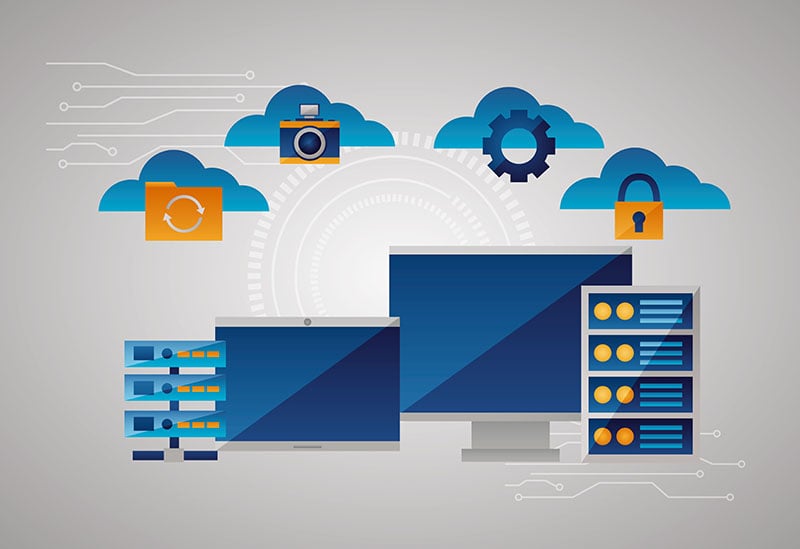
Hybrid Cloud platforms have reshaped the technology landscape for business analytics as an optimal tradeoff solution for cost, performance, and security of analyzing large volumes of sensitive business information in real time. The hybrid cloud computing model includes private and public cloud and on-premise data center infrastructure. The
computing environment is orchestrated as a hybrid cloud model that allocates resources across different IT workloads and data assets depending on the business use case, security sensitivity, and SLA dependability requirements.
Analytics use cases that process large volumes of anonymous data may be stored and analyzed in public cloud resources to reduce cost. Security-sensitive business information and apps subject to high availability SLA standards may run on dedicated private cloud systems or expensive on-premise data center systems to meet stringent compliance regulations.
The hybrid cloud model for data analytics helps organizations overcome the technical limitations of an individual cloud computing model. While public cloud services are less expensive and highly scalable, the pooled computing resources accessed over the Internet offer limited security and performance. The private cloud model is more costly, but the dedicated resources guarantee high availability. On-premise infrastructure resources ensure that mission-critical apps and services and sensitive business information stays within the geographic, physical as well as logical boundaries of the organization's internal IT network. This model is essential for security-sensitive analytics use cases in highly regulated financial, healthcare, and defense industry verticals. For these reasons, organizations are adopting the hybrid cloud model to build modern data lake platforms for advanced data analytics use cases.
Enterprise Data Lakes to Remove Barriers
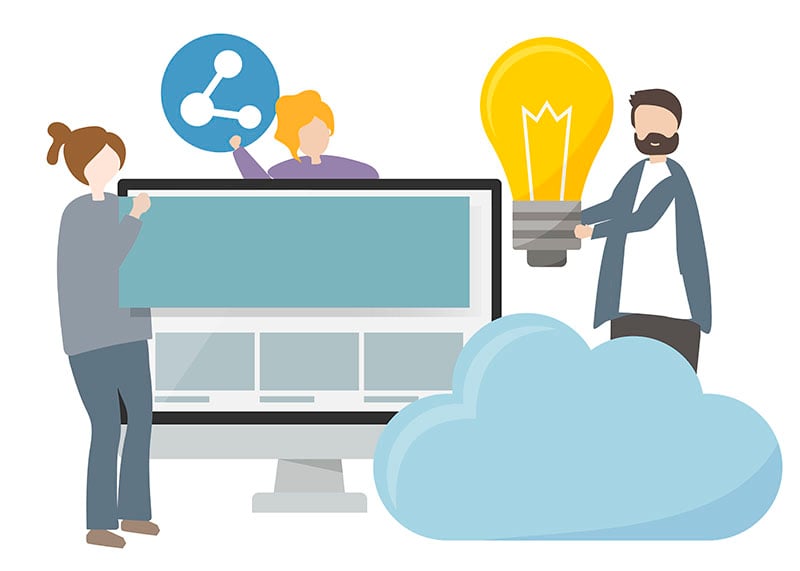
Data lake technologies offer better visibility into data assets, improve data analysis control, and reduce the cost associated with the end-to-end analytics pipeline. This is important for analytics use cases that process large volumes of unstructured real-time data streams at scale – in a traditional data warehouse setting, schema-on-write ETL transformation slows down the analytics process. Suppose multiple formats are required for the same enterprise data assets. In that case, the cost of storing duplicate versions on public cloud storage services such as Amazon S3 can also increase exponentially.
On the other hand, a data lake implementation offers the flexibility to enforce a holistic and unified data governance and access control strategy on the centralized data repository. Data consumers are adequately segregated, and a diverse range of compliance and governance controls and mechanisms can be enforced without introducing duplicated enterprise data assets in silos, manual pre-processing, or anti-democratization of data assets.
How do you modernize your existing data warehouse systems?
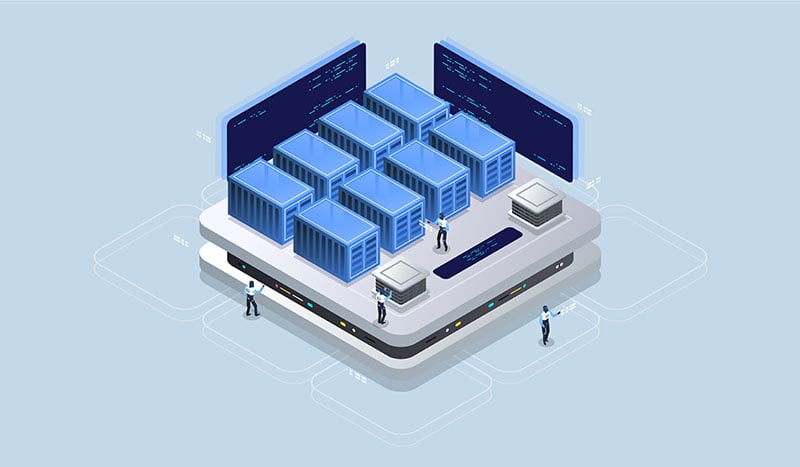
The idea of a modern data platform such as a data lake is to unify data management activities, ranging from data orchestration to querying, reporting, and analysis. Using automation tools to simplify data movement can be a starting point but eventually limit the scalability of the data platform as more data sources are acquired and more third-party analytics services are integrated into the system.
The holistic modernization strategy for your data warehouse must account for three critical perspectives:
- A business perspective that aims to achieve the business goals of a modern data-driven enterprise.
- A technical standpoint that aims to meet the technical performance of highly scalable, secure, and performance-sensitive analytics use cases.
- An operational perspective that removes the process bottlenecks in your digital transformation strategy.
If you are looking forward to building a comprehensive modern data strategy.