CASE STUDY
Smart Factory - Control And Command Center
Mactores helped Hewlett Packard transform its factory analytics and operations using real-time data and machine learning to enhance operational efficiency by 40% and identify manufacturing anomalies to improve yield by 30%. Over 60 active data points now support real-time dashboards & analytics capabilities solving their chip yield, chip quality, and pilferage challenges.
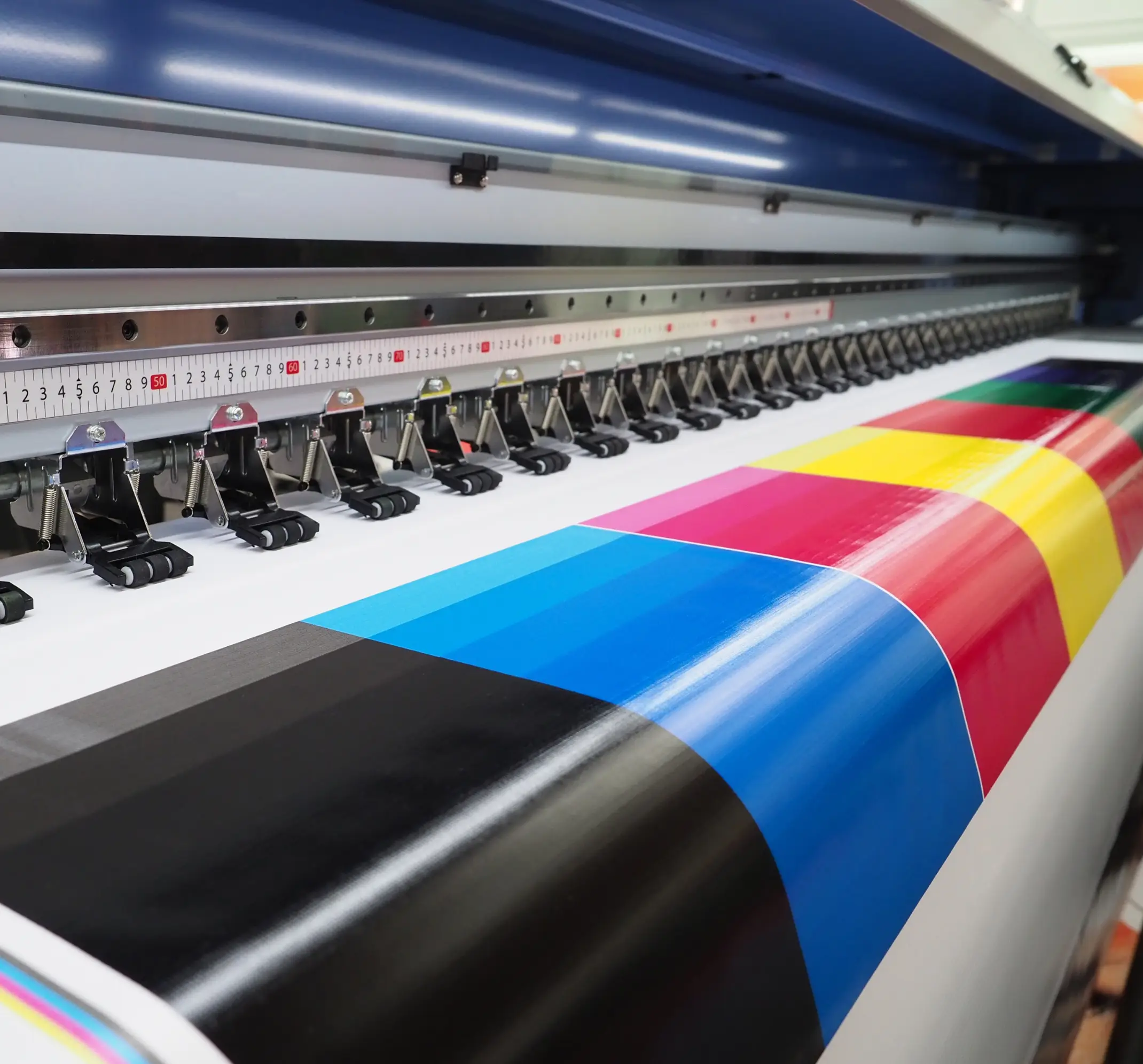
About
The Customer
HP Inc. develops personal computers (PCs), printers, related supplies, and 3D printing solutions. As a leading manufacturing company with 30 Million daily chips being personalized and 60+ active data points to track, it faced the challenge of managing the high throughput of the chip yield data and yield quality.
Specific to the Ink Division, the company has benefitted from strong office and home printing businesses. Now that Hewlett-Packard has already extended inkjet technology beyond printers to related devices like copiers and fax machines, the most ambitious move is the $35 billion photography market with an inexpensive digital camera and a new line of printers produce photo-quality color prints.
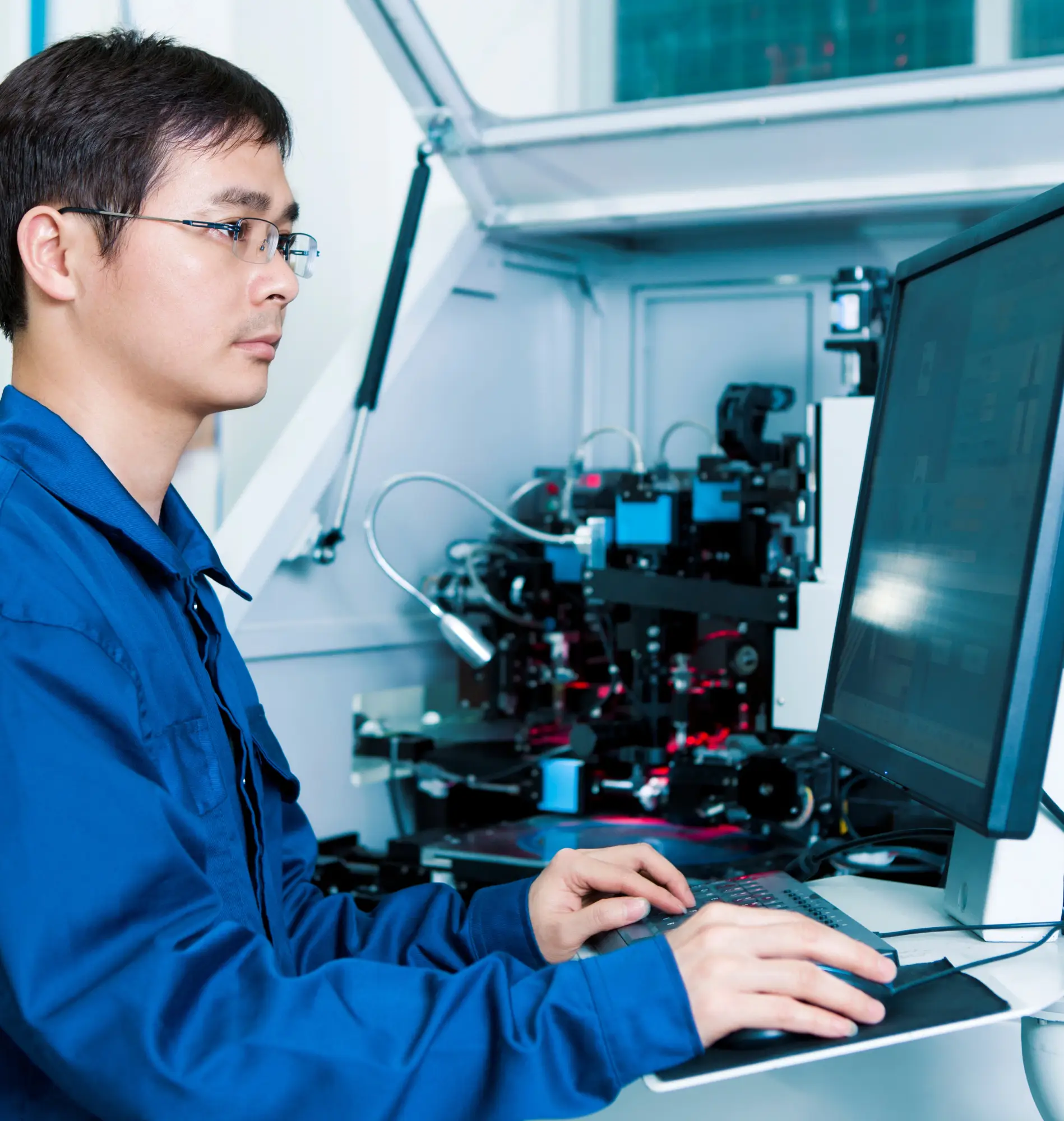
“The Mactores team executed precisely, and their measurable KPIs kept us aligned during all critical data delivery and system design. They enabled us to remain agile and deliver business objectives on time.”
Customer Situation
HP was experiencing a lack of centralized end-to-end inventory visibility as multiple systems supplied data with different data sources. Solving this was critical for the key stakeholders, which include HP Planning, HP Procurement, Material Supplier, HP Work Center, and Contract Manufacturers.
Accurate inventory tracking is essential for accurate demand planning and procurement, reducing bottlenecks in production, production quality, and pilferage.
Tracking the yield percentages (%) at each stage in the process helped identify material quality issues earlier (via alerts to the right owners) and can be fed back up the manufacturing chain to the supplier.
Cycle time tracking is key to identifying inefficiencies and driving continuous process improvements.
Our Approach
Mactores data engineering team extensively assessed HP’s manufacturing process to understand the datasets and data acquisition processes. The assessment results helped the Mactores manufacturing domain experts work with the data engineers and scientists to build a real-time data acquisition, cleaning, and exploration process.
After initial data acquisition and cleaning of historical data, the Mactores data scientists performed various machine learning experiments to identify features and models to solve HP’s business problems. To solve HP’s real-time processing and in-stream data analytics, Mactores recommended Amazon MSK and Amazon Kinesis Firehose to ingest in the data lake using AWS Lambda.
Business Outcomes
The modeling system by Mactores predicts the possibility of pilferages at each level in the manufacturing process, thereby performing a 30% improved yield prediction. Material/Manufacturing quality detection helped HP improve quality and reduced post-manufacturing defects by 40%. By analyzing these data points in real-time, HP had better control over the manufacturing operations, providing them with a highly scalable modern Control and Command Center.
Mactores team effectively integrated with Amazon Athena to give the manufacturing company a single window to explore their data to help them create new models to achieve business objectives with Machine Learning by building data models for data analytics using Amazon SageMaker and Tableau.
Technical Outcomes
With real-time data analytics, HP’s data engineering team could now derive better insights into the manufacturing processes and operations. The Mactores data science team worked with the HP team to build anomaly detection models using Apache SparkML while also solving the material quality issue (Manufacturing Diseases) Classification using Convolutional Neural Networks using Tensorflow.
To help HP identify real-time micro-batch processing and in-stream data processing, Mactores recommended Amazon MSK and Amazon Firehouse, and Amazon S3. At the same time, Amazon EMR was used for data pipelines and micro batching using Apache Spark Streaming.
.
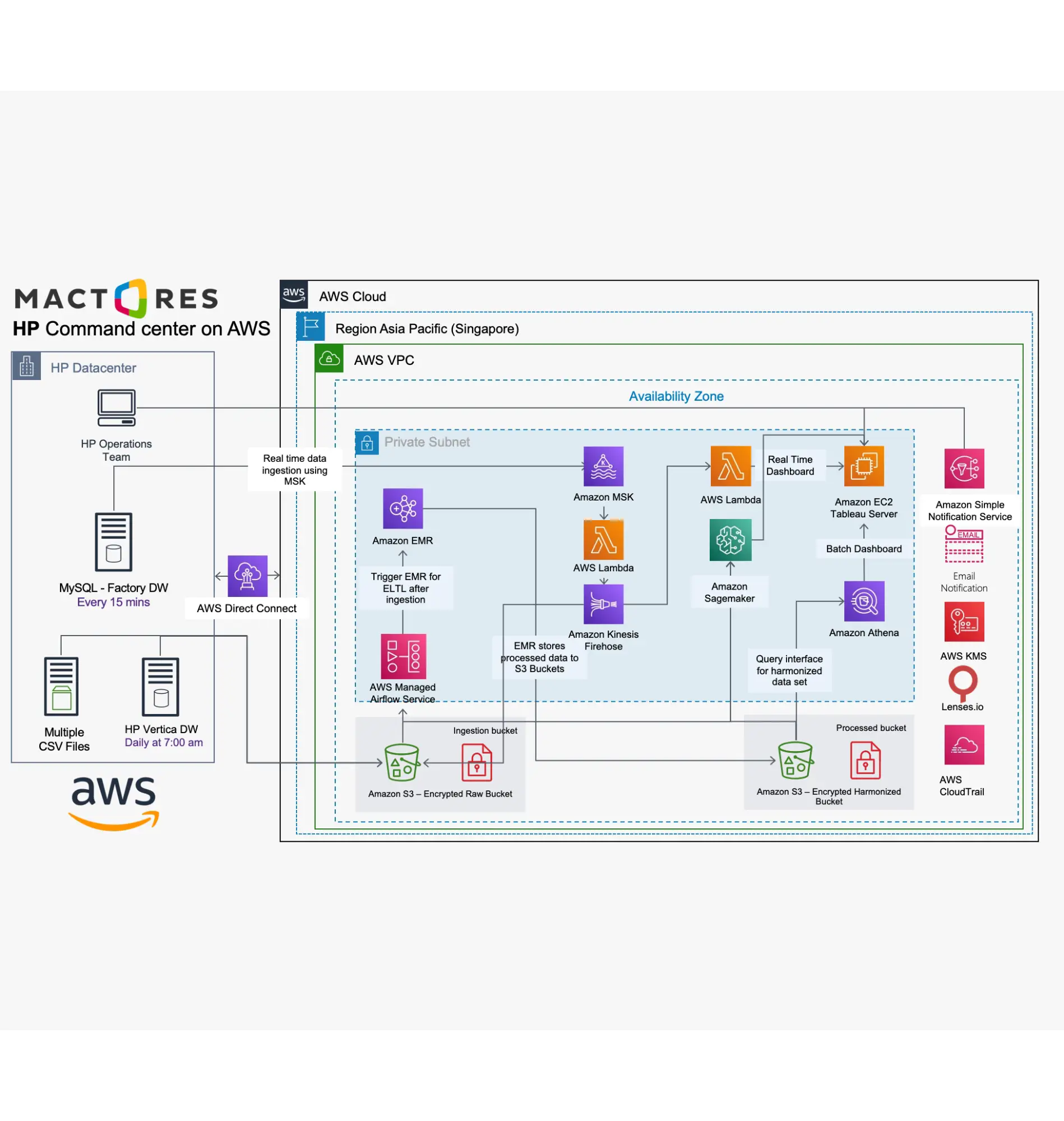
Reference
Architecture
Services Delivered by Mactores
- Material quality issue (Manufacturing Diseases) Classification using Convolutional Neural Networks using Sagemaker.
- AWS Architecture Consulting, Data Modelling, Model fitting, and Model Optimization
- Implement Mactores Aedeon data lake and data pipelines
- Migrate on-premise Apache Kafka to Amazon MSK with Lenses.io for observability
- Build models for data analytics using Amazon Sagemaker, Tableau, and Plotly
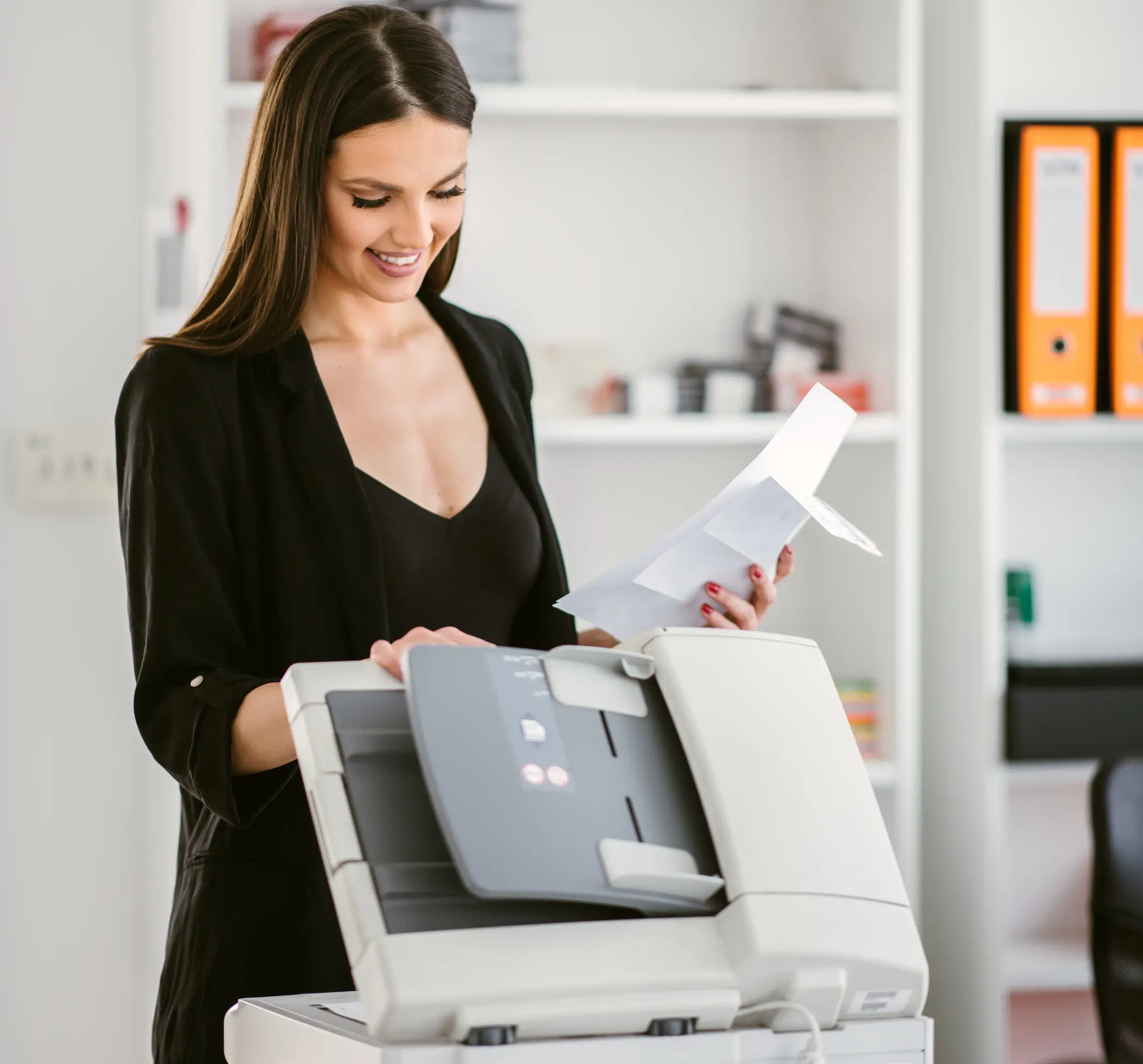
Getting
Started
Mactores conducted an immersive onsite workshop, providing the key stakeholders with a deep dive into their current state. Once the HP team was aligned, the Mactores data team conducted an ideation session, helping HP see its path forward.
This workshop led to a Proof of Concept, lasting just 4 weeks, providing the team quick wins to socialize internally.
By seeing the new solution applied to their environment, the stakeholders came together quickly on the right solution. With a clear path forward, the Mactores team partnered with HP to deploy their modern Control and Command Center.