CASE STUDY
AI-backed operational data lake for Synaptics
Synaptics was challenged to operate and manage an EDA workload for multiple designs and testing teams using an HPC solution. Mactores Data Lake and AI solutions improved their design jobs' efficiency by 75% and the throughput of the HPC solution by 40%.
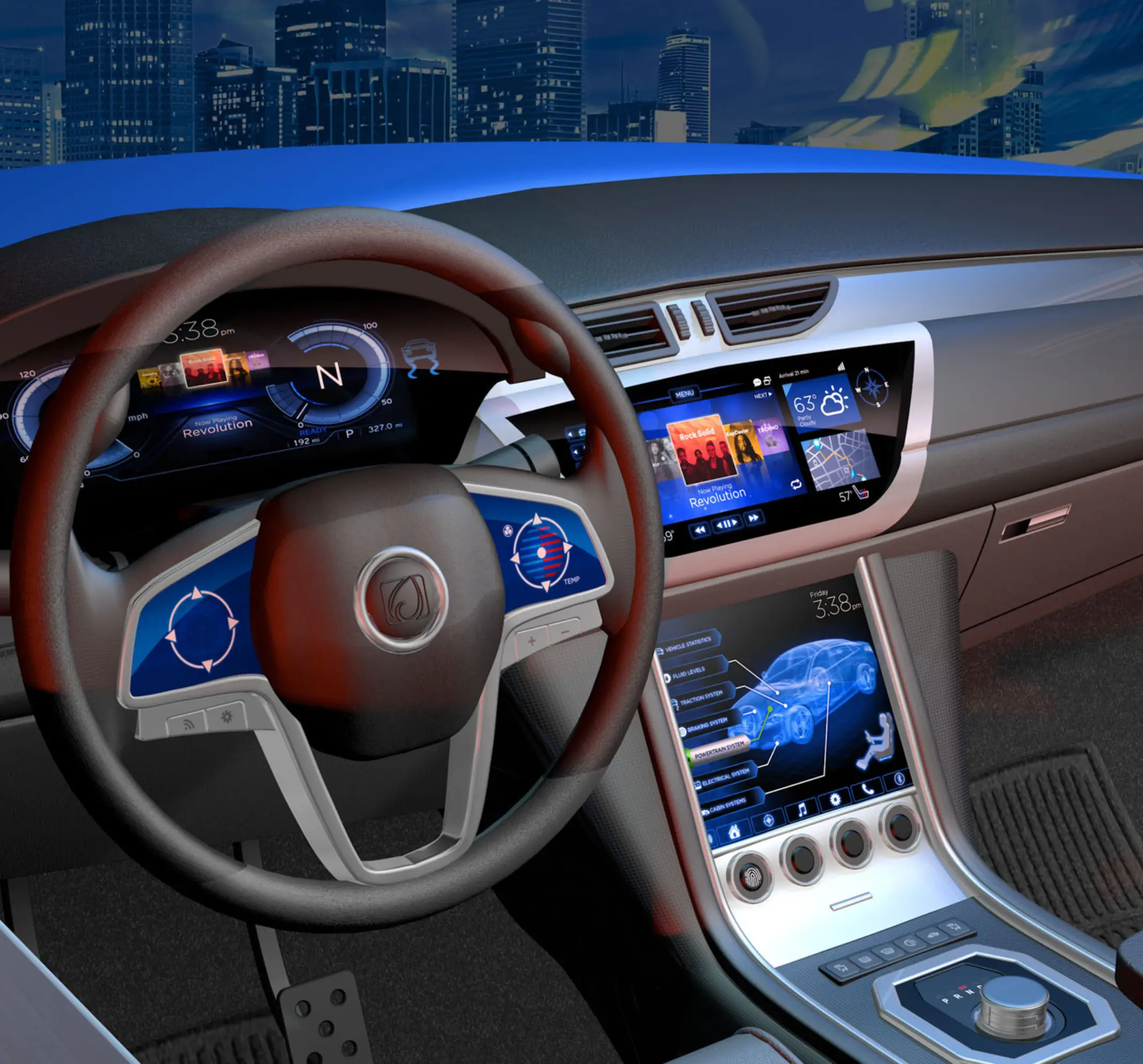
About
The Customer
Synaptics is a developer of human interface hardware and software, including touchpads for computer laptops; touch, display driver, and fingerprint biometrics technology for smartphones; and touch, video, and far-field voice technology for smart home devices and automotive.
As a silicon manufacturing and design company, HPC (High-Performance Compute) solutions like IBM® LSF™ process their EDA(Electronic Design Automation) jobs across 300-900 servers, distributed in multiple clusters.
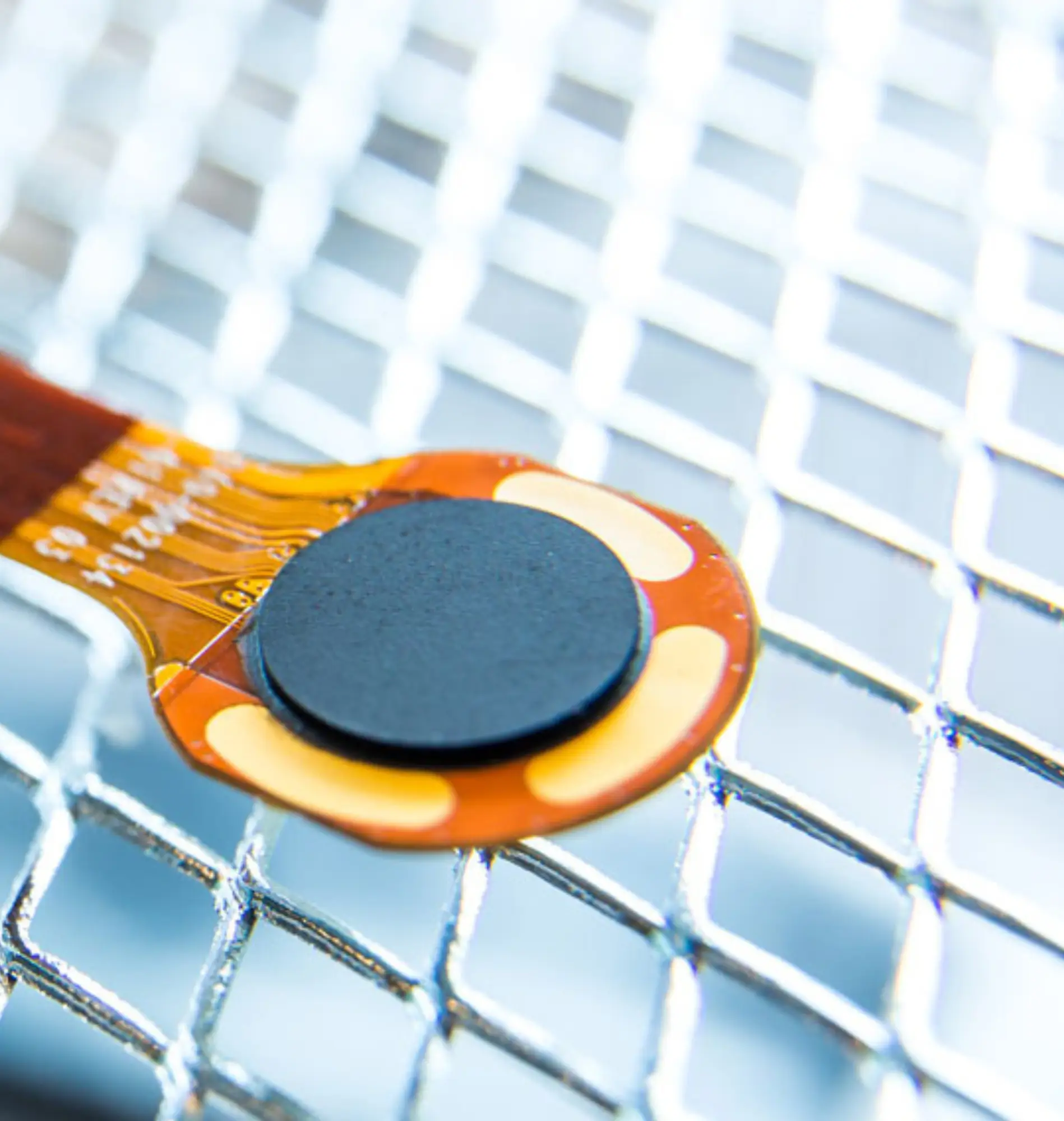
“We were able to optimize and confidently re-architect our HPC Clusters for better resource utilization, enhanced job performance, improve the throughput of one of our largest EDA clusters by up to 40%, and improve queue contention related to jobs having 75% lesser ‘wait/pending’ times using smart queues. The AI-driven forecasting model will help us predict future resource requirements and accelerate our cloud journey.”
Customer Situation
Synaptics EDA (Electronic Design Automation) Team had challenges understanding resource utilization of their IBM® LSF™ cluster for various projects and jobs executed by multiple teams across six global locations.
Additionally, the CAD (Computer Aided Design) team at Synaptics was challenged by forecasting resources and licenses for each new project the EDA team would need to complete. This meant the EDA team would get inconsistent performance for the same or similar jobs on LSF clusters, significantly affecting project execution timelines.
Therefore, all business units dependent on the EDA team for project execution did not have the proper resource utilization reporting and chargeback model for their LSF clusters.
Our Approach
To help the Synaptics team, Mactores built an operational data lake based on the IBM® LSF™ and RTM™ data to predict job runtime and resource utilization. Mactores also developed the model to forecast resources and license utilization for EDA jobs for new projects. Athena and QuickSight-based dashboards were created for CAD and EDA teams to perform data analytics for IBM® LSF™ cluster utilization.
Predicting Job runtime and resource utilization enabled Synaptics to implement Smart Queues for the IBM® LSF™ cluster, allowing optimum cluster utilization and consistent performance.
Business Outcomes
Synaptics EDA team achieved 75% improved efficiency of the BM® LSF™ cluster. The business units now execute projects 10x -12x faster with smart queues. The dashboards have allowed IT, CAD, and EDA teams to analyze the bottlenecks in the EDA jobs daily to optimize the license and hardware resources based on project priorities providing significant savings.
The new AI-backed forecasting model helps Synaptics predict resource requirements, and expand the cluster to AWS on-demand infrastructure.
Technical Outcomes
The skew in the cluster queue was predicted using “job execution time” based on time series forecasting models backed by Amazon Sagemaker and enqueuing the jobs in the queues with sufficient resource availability. The Mactores AI team recommended a new cluster configuration based on the classification of jobs. Multiple queues were recommended based on the estimated execution time to allocate a fair share of the cluster resources.
The new queuing mechanism ensured that jobs were processed faster and were more manageable while distributing computing resources for complex jobs in separate queues.
.
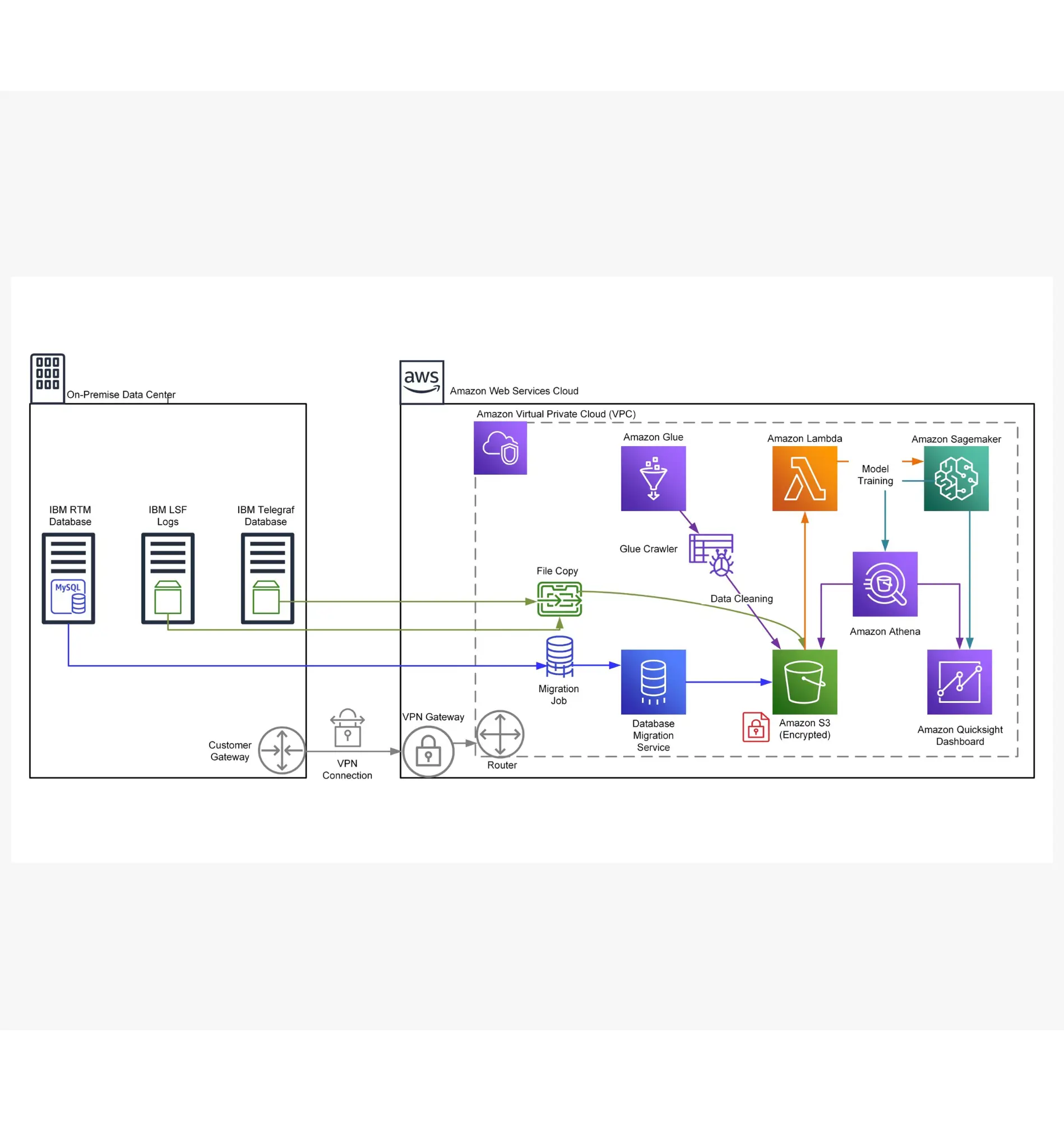
Reference
Architecture
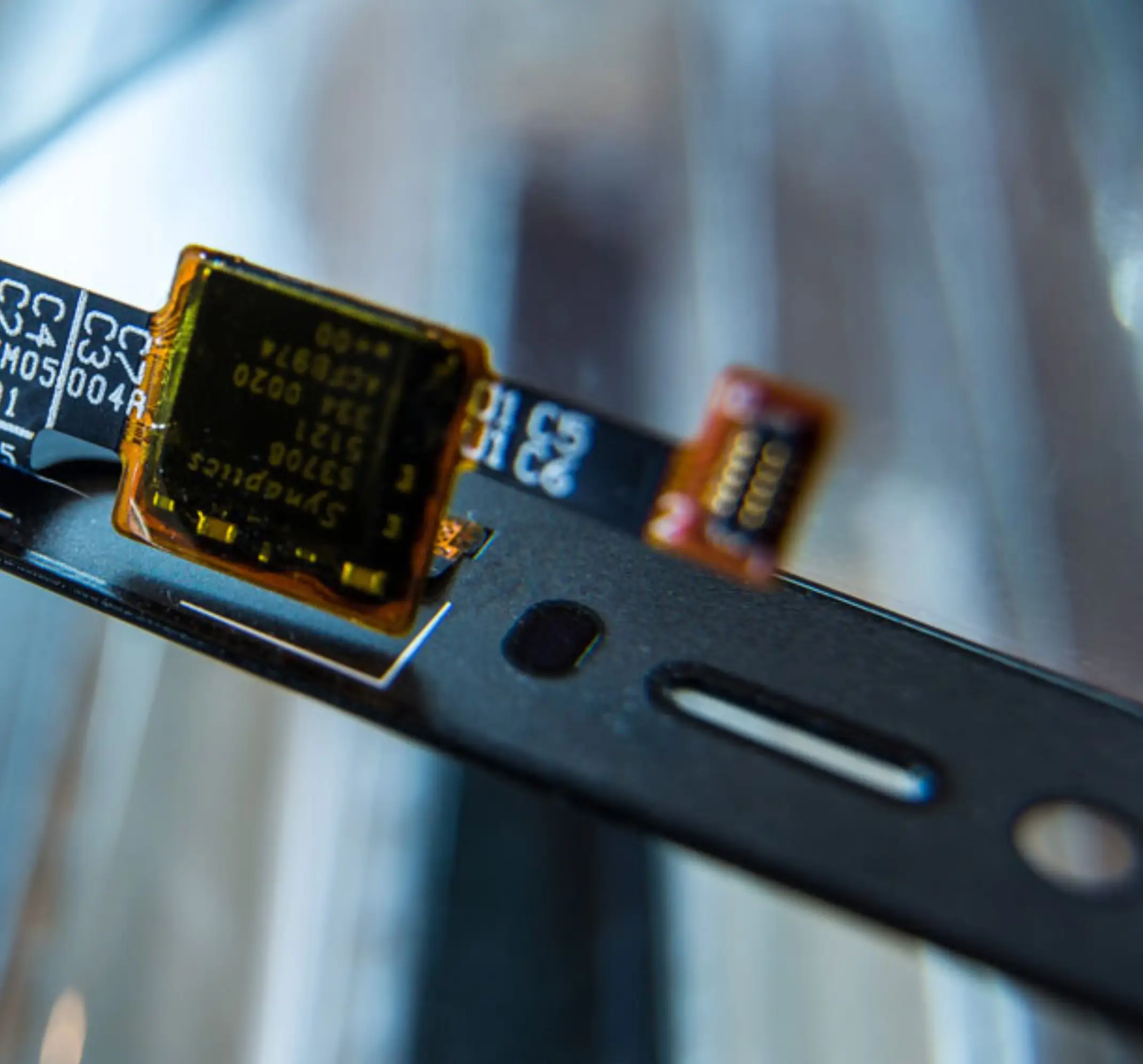
Getting
Started
Mactores conducted an immersive workshop, providing the key stakeholders with a dive deep into their current state. Once the Synaptics team was aligned, the Mactores data team worked on an ideation session, helping the Synaptics team see their path forward.
This workshop led to a Proof of Concept, lasting just 4 weeks, allowing the team to experience quick wins by seeing the new solution applied to their environment, creating the buy-in for all the key stakeholders. With a clear path forward, the Mactores team partnered with Synaptics to begin their transformation.