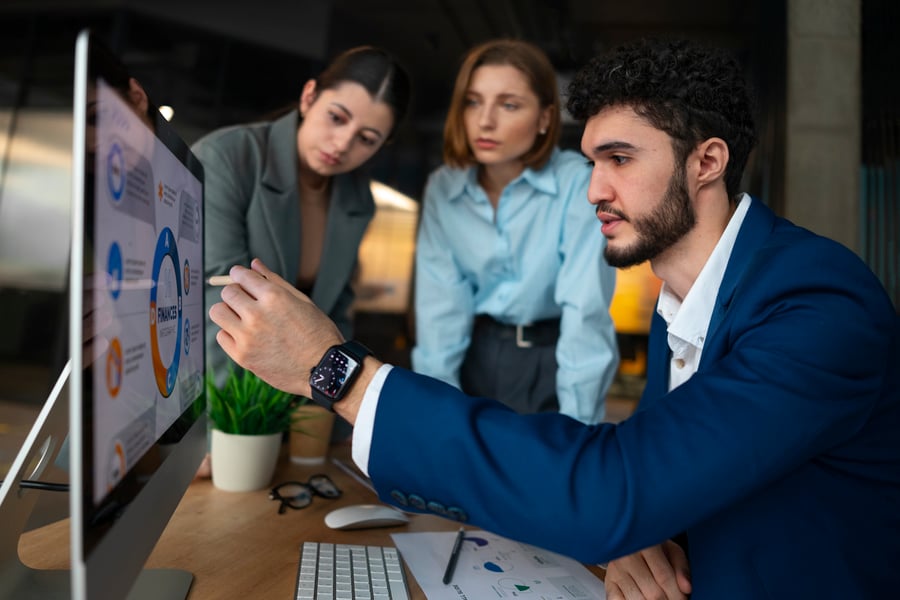
Critical Principles of Automated MLOps
- Continuous Integration and Continuous Delivery (CI/CD) for ML: Treat ML models like software code, enabling rapid iteration and testing through automated pipelines.
- Version Control and Model Governance: Track and manage different versions of models, ensuring consistency and regulatory compliance.
- Automated Monitoring and Performance Tracking: Continuously monitor model performance in production, identifying drift and alerting teams to potential issues.
- Collaboration and Tooling: Promote collaboration between data scientists, engineers, and IT teams through shared tools and platforms.
Automating repetitive tasks and streamlining workflows, automated MLOps deliver significant efficiency gains. Data scientists can automate MLOps to reduce development time and costs. Automating data preprocessing, training pipelines, and testing will free up data scientists for more strategic activities.
In addition, you will witness consistent model development and deployment, reduced errors, and quick identification and fixes of issues. In this way, automated MLOps are pivotal in optimizing operational workflows.
Advantages of Automation in MLOps
Faster Development Cycle
Automated MLOps can accelerate the development cycle to a great extent. By automating repetitive tasks and workflows in a development cycle, for example:
- Data Preprocessing: Use automated tools to handle data cleaning, normalization, and transformation, ensuring data is ready for model training without manual intervention.
- Feature Engineering: Automated MLOps can analyze data patterns, detect correlations, and generate new features based on predefined rules or machine learning algorithms.
- Model Training: MLOps automation can automate selecting, training, and optimizing machine learning models, reducing the need for manual parameter tuning.
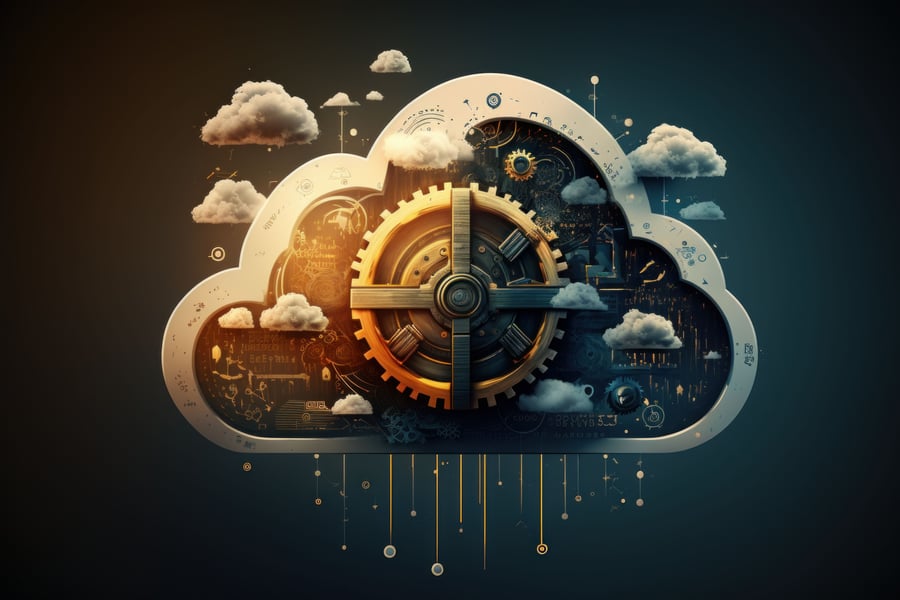
Rapid Prototyping
Automated MLOps is a playground for data scientists, enabling them to test and refine ideas rapidly.
- Agile Infrastructure for Experimentation: Utilize cloud-based platforms and pre-built components to spin up new models in minutes, fostering exploration and innovation.
- Frictionless Iteration and Testing: Quickly build and test different model variants through automated pipelines, validating ideas and identifying the best solutions.
- A/B Testing Made Easy: Seamlessly deploy and compare various model versions in production, A/B testing features and optimizing performance based on real-world data.
Nurturing Innovation Mindset within Businesses
Automated MLOps is not just about efficiency; it's about unlocking the true potential of your data science team. By removing the manual burden from routine tasks, MLOps will act as a catalyst for innovation.
- Empowered Data Scientists: Freeing them from repetitive tasks allows them to focus on creative problem-solving and explore new techniques.
- A culture of experimentation: A continuous learning environment encourages risk-taking and exploration, driving long-term business growth and adaptation.
- ML for all: Enable non-technical teams to leverage pre-built models and automated workflows, promoting broader participation in the innovation process.
Real-world Examples and Case Studies of Automated MLOps
- Predictive maintenance identifies potential equipment failures before they happen, preventing costly downtime.
- Optimized production lines adapt to real-time demand fluctuations, maximizing efficiency and output.
- Personalized product recommendations based on factory sensor data improve customer satisfaction and boost revenue.
Also, many businesses are already reaping the benefits of automated MLOps. For example, consider these compelling cases:
- JPMorgan Chase uses automated MLOps to identify real-time fraudulent transactions, reducing financial losses and data breaches.
- Uber: They deploy and monitor complex pricing models in real time, optimizing pricing based on demand and location.
Automated MLOps Integration into Existing Workflows
- Identify Key Areas for Automation: Pinpoint bottlenecks and repetitive tasks in your current ML workflow. You can start by assessing their existing processes and identifying areas that can benefit from automation. Whether it's data preprocessing, model training, or deployment, a phased approach to integration ensures a smooth transition without disrupting ongoing operations.
- Select the Right Tools and Technologies: Choose tools that align with your specific needs and infrastructure, considering scalability and integration capabilities. You should also consider your existing technology stack and cloud environments.
- Address Cultural and Organizational Changes: Encourage collaboration between data science and IT teams, ensuring everyone understands the benefits and responsibilities of automation. Implement a phased approach, starting with small pilots and gradually scaling up. Make sure to provide ongoing support and training to ensure smooth adoption.
In the meantime, track the impact of automation on key performance indicators (KPIs) – development time, model performance, etc. Remember that it's an ongoing process – continually evaluate, adapt, and refine your approach to maximize the benefits of automated MLOps and unlock the full potential of your data and teams.
Conclusion
The impact of Automated MLOps on business efficiency and innovation cannot be overstated. As we look toward the MLOps automation trends like explainable and responsible AI and MLOps for edge computing, the message is clear: the future of business competitiveness lies in embracing MLOps automation. It's time to adopt automation and stay ahead in the AI race. And the journey towards a more efficient and innovative future begins with Automated MLOps. Would you be ready to simplify your machine-learning journey? Let's talk now for a personalized consultation on how to use Automated MLOps for your business success.